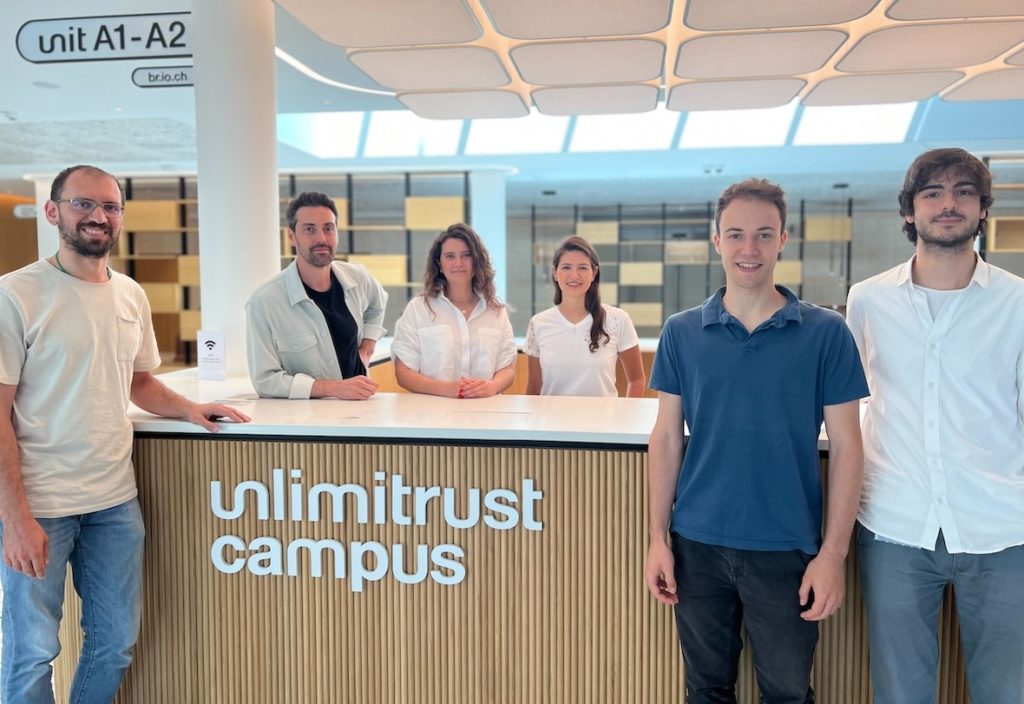
EPFL unveils a modular AI model for diverse data analysis
12 March 2024
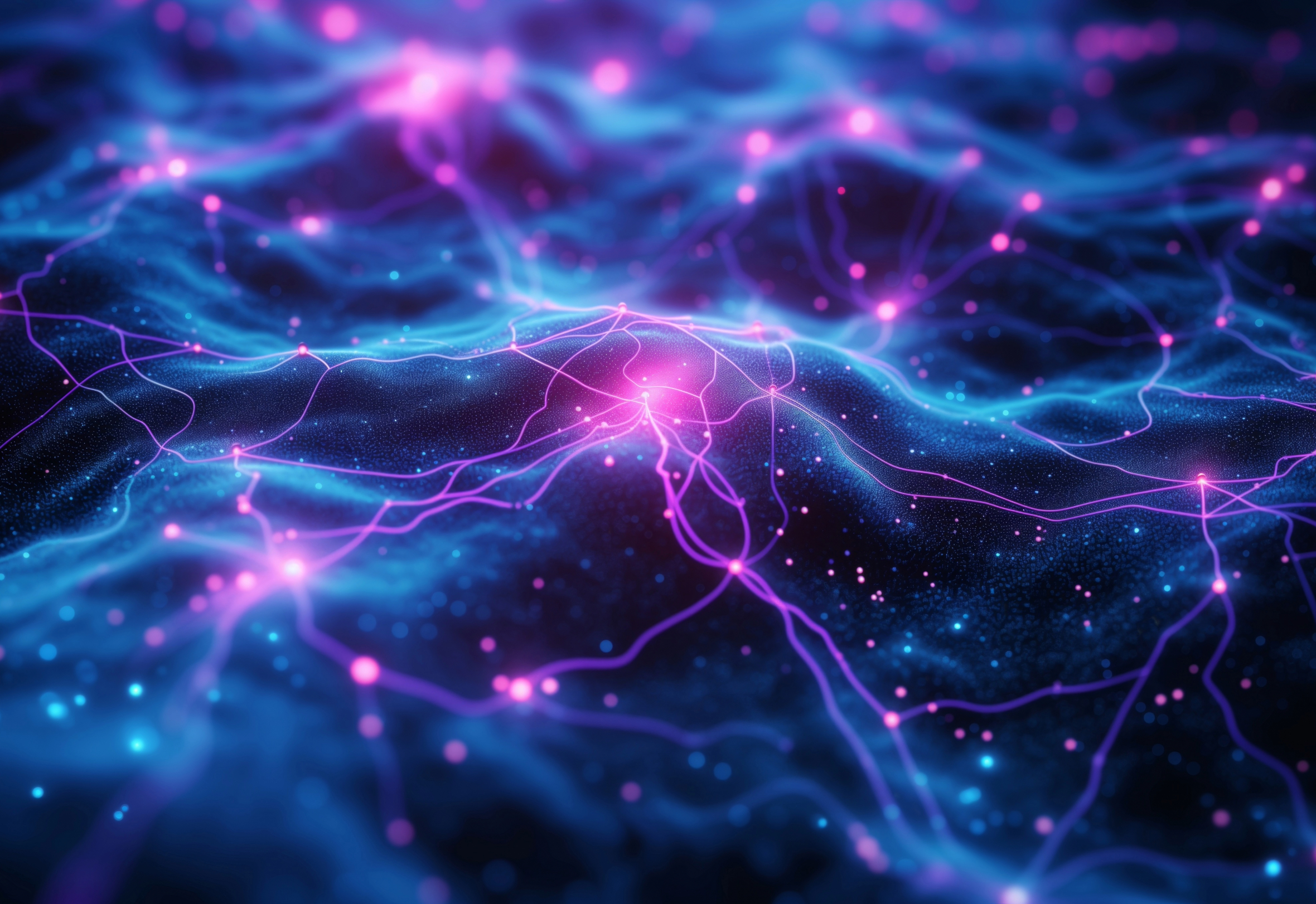
Researchers at the EPFL have unveiled a groundbreaking machine learning model that could herald a new era in the versatility and application of artificial intelligence.
In contrast to Large Language Models (LLMs) like OpenAI’s ChatGPT, which are built on vast textual databases, EPFL’s new model, dubbed MultiModN, is tailored for the next-generation Multimodal Models (MMs) sphere. These MMs excel by learning from a variety of data types, yet smaller-scale models face hurdles, notably in handling non-random missing information without absorbing biased patterns. MultiModN emerges as a pioneering solution to this challenge.
Developed under the stewardship of Professors Mary-Anne Hartley and Tanja Käser, MultiModN is structured around numerous smaller, self-sufficient modules. This architecture allows for the selection and sequencing of modules based on available data, culminating in a highly adaptable and interpretable model. Tested across ten real-world tasks, including medical diagnosis support and academic performance prediction, MultiModN has demonstrated its robustness and interpretability, especially in scenarios marred by Missing Not At Random (MNAR) data.
The model’s inaugural application focuses on enhancing clinical decision-making in resource-constrained environments. MultiModN’s inherent flexibility and bias-resistant learning mechanism make it ideally suited for healthcare settings where data gaps are prevalent due to economic or redundancy factors. By leveraging real-world clinical data without inheriting its biases, MultiModN aims to support medical personnel by adjusting its predictions based on the available inputs.
Aligning machine learning advancements with societal needs
Beyond publication, efforts are underway to translate MultiModN from laboratory to practical use, with collaborations between EPFL, Lausanne University Hospital (CHUV), and University Hospital Bern, focusing on diagnosing pneumonia and tuberculosis in low-resource regions. A comprehensive data collection initiative is also in progress, aiming to train MultiModN with real-world, multimodal data reflective of conditions in low-resource settings.
This development represents EPFL’s continued commitment to aligning machine learning advancements with societal needs. Alongside Meditron, another top-performing open-source LLM for clinical decision support, MultiModN embodies EPFL’s vision for responsible AI that fosters technological innovation across various societal sectors, underscored by the ethos of the new EPFL AI Center.